The Psychology Behind AI Recommendations: Understanding Customer Behavior Through Data
Imagine AI recommendations as the silent observers of a bustling marketplace, deciphering the intricate dance of customer choices.
As you navigate the realm of online shopping, have you ever wondered how these tailored suggestions seem to anticipate your desires?
The fusion of psychology and technology in AI recommendation systems unveils a captivating realm where data illuminates the path to understanding consumer behavior.
Key Takeaways
- Data-driven insights are crucial for personalized AI recommendations.
- Personalization enhances user engagement and satisfaction.
- Emotional intelligence plays a significant role in predicting consumer reactions.
- Ethical considerations, including data privacy and fairness, are essential in AI recommendation systems.
The Role of Data in AI Recommendations
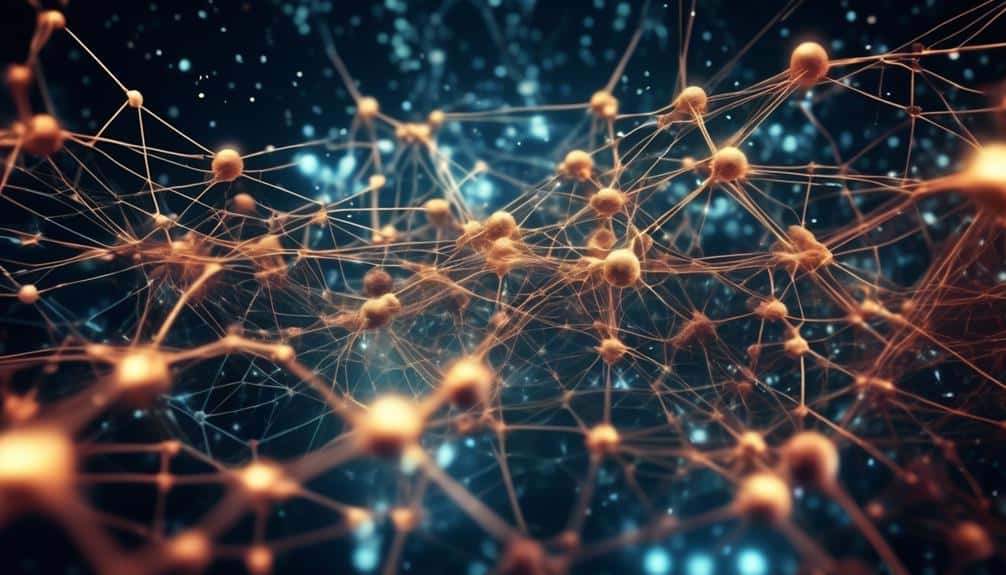
To optimize AI recommendations, data plays a crucial role in tailoring personalized suggestions to individual customers. Through meticulous data analysis, AI algorithms can delve into user behavior patterns, preferences, and interactions with the platform. By scrutinizing vast amounts of data, AI systems can identify correlations and trends that mightn't be immediately apparent to human observers.
Data analysis forms the bedrock of AI recommendations, enabling the system to understand user behavior on a granular level. By tracking user clicks, views, purchases, and other interactions, AI can create detailed user profiles that inform the recommendations presented to each individual. This personalized approach enhances user satisfaction and engagement with the platform.
In essence, the effectiveness of AI recommendations hinges on the quality and depth of data analysis conducted. The more comprehensive the understanding of user behavior, the more accurate and relevant the recommendations become. Data-driven insights empower AI systems to adapt in real-time to changing user preferences, ensuring a dynamic and responsive user experience.
Understanding User Preferences With AI
To understand user preferences with AI, you need to focus on personalized content recommendations and behavioral data analysis.
By leveraging AI algorithms to analyze user behavior, you can gain valuable insights into individual preferences and tailor recommendations accordingly.
This approach enables a more targeted and effective strategy for engaging customers based on their unique interests and actions.
Personalized Content Recommendations
Understanding user preferences with AI allows for the creation of personalized content recommendations tailored to individual needs and interests. This approach leverages data-driven recommendations to enhance user engagement and align with consumer behavior.
- Content Personalization and User Engagement:
- AI algorithms analyze user interactions to deliver customized content.
- Tailored recommendations increase user satisfaction and retention.
Behavioral Data Analysis
Analyzing user behavior through AI-powered data insights enables the generation of targeted recommendations aligned with individual preferences and interests.
By delving into consumer behavior patterns, data analysis provides a detailed understanding of how users interact with content, make choices, and engage with products or services.
Through behavioral data analysis, AI algorithms can identify trends, preferences, and correlations that may not be immediately apparent, allowing for the creation of personalized recommendations that resonate with each user.
This meticulous examination of consumer behavior through data analysis serves as the foundation for enhancing user experience, increasing engagement, and ultimately driving conversion rates.
Leveraging AI to interpret behavioral data empowers businesses to tailor their offerings to meet the unique needs and desires of their customers efficiently and effectively.
Personalization in Customer Experience
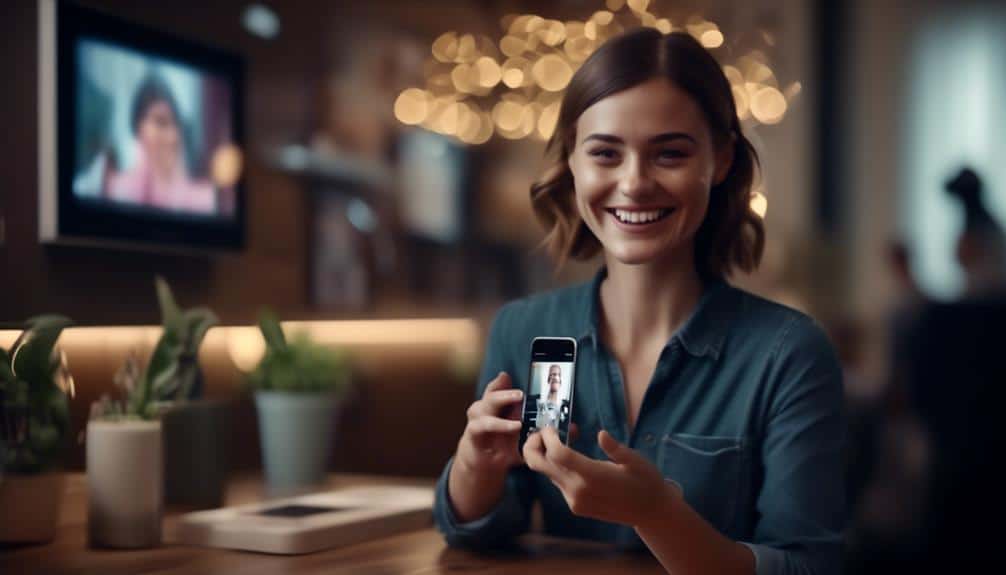
You need to focus on tailored user experiences, customized product recommendations, and behavioral data analysis when looking at personalization in customer experience.
These points are crucial for enhancing customer satisfaction and driving engagement.
Tailored User Experiences
Incorporating personalized recommendations based on user preferences enhances the overall customer experience and fosters customer loyalty. Tailored user experiences are crucial for improving user engagement and customer satisfaction.
- User Engagement:
- By offering personalized content, users are more likely to stay engaged with your platform.
- Tailoring interactions based on user behavior boosts active participation.
Personalization creates a seamless and intuitive journey for customers, making them feel understood and valued. By analyzing user data and providing customized suggestions, businesses can significantly enhance user satisfaction and encourage repeat interactions. This tailored approach not only increases customer loyalty but also drives higher retention rates and boosts overall revenue.
Customized Product Recommendations
Customized product recommendations play a pivotal role in enhancing the personalization of the customer experience, driving engagement, and fostering brand loyalty. Product customization taps into consumer psychology, catering to individual preferences and behaviors. Recommendation algorithms analyze user data to offer tailored suggestions, aligning with each customer's unique needs and desires.
Behavioral Data Analysis
Analyzing behavioral data is essential for enhancing personalization in the customer experience, building on the foundation of customized product recommendations. By delving into consumer psychology, businesses can uncover valuable insights that drive decision-making. Through data-driven insights, patterns emerge, revealing preferences, tendencies, and purchasing behaviors.
- Consumer Psychology Insights:
- Understanding the emotional triggers behind consumer actions.
- Identifying subconscious motivations that influence purchasing decisions.
This analytical approach allows companies to tailor their offerings to individual preferences effectively, creating a more engaging and personalized experience for customers. Embracing behavioral data analysis not only improves customer satisfaction but also boosts brand loyalty by demonstrating a deep understanding of consumer needs.
Behavioral Insights Driving AI Recommendations
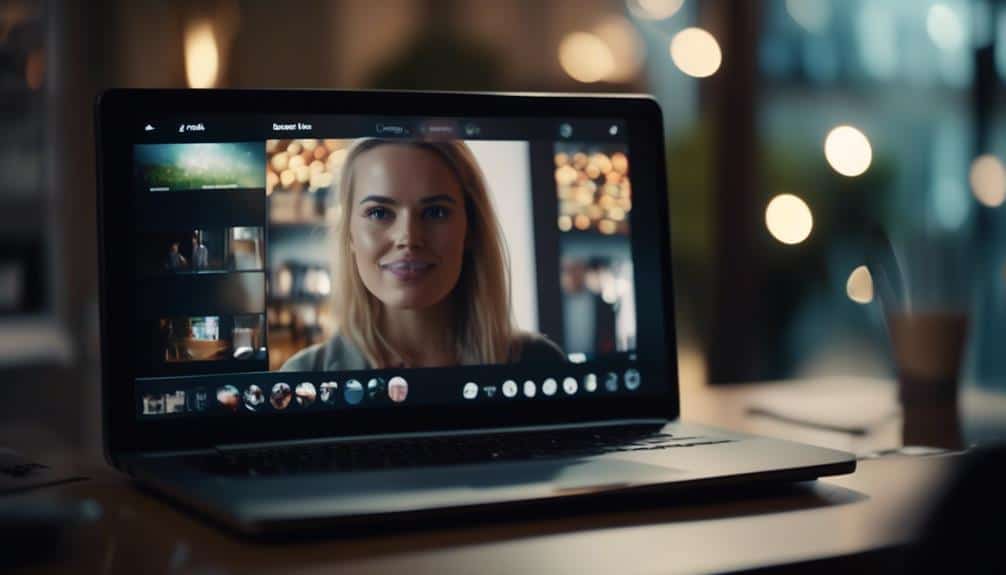
Understanding customer behavior is pivotal in driving AI recommendations that resonate with individual preferences and needs. By analyzing emotional intelligence and decision-making processes, AI can offer personalized recommendations that cater to the unique psychological aspects of each customer.
Emotional intelligence plays a crucial role in predicting consumer reactions to different stimuli, enabling AI systems to suggest products or services that align with their emotional states. Furthermore, by understanding decision-making processes, AI can anticipate how customers evaluate options and make choices, allowing for more accurate and effective recommendations.
These behavioral insights empower AI algorithms to not only recommend relevant items but also consider the emotional and cognitive factors that influence customer decisions. Incorporating these insights into AI recommendation systems enhances their ability to connect with customers on a deeper level, ultimately improving user satisfaction and driving business success through tailored suggestions that align with individual preferences.
Impact of Emotions on Recommendations
Emotions significantly influence the recommendations provided by AI systems, shaping the personalized offerings based on individual psychological responses. Emotional influence plays a crucial role in the decision-making processes of AI algorithms, impacting the suggestions presented to users.
- Positive Emotions: When a customer exhibits happiness or satisfaction, the AI system may prioritize recommending products or services that align with their current positive emotional state. This could lead to increased customer loyalty and higher satisfaction levels with the recommendations received.
- *Imagine*: A user smiles after making a purchase and receives suggestions for complementary items, enhancing their overall shopping experience.
- Negative Emotions: Conversely, if a user displays frustration or disappointment, the AI system might adjust recommendations to offer solutions that address the underlying issues causing these negative emotions. By addressing customer concerns promptly, the system can potentially improve user retention and brand perception.
- *Imagine*: A customer frowns while browsing, triggering the AI to suggest troubleshooting guides or alternative products to alleviate their frustration.
Cognitive Biases in AI Suggestions
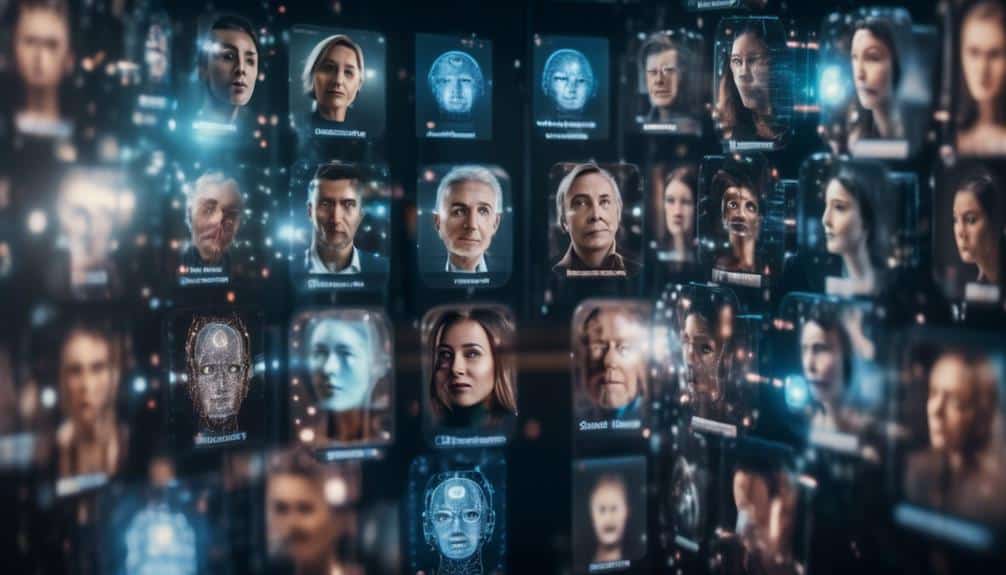
In the realm of AI recommendations, cognitive biases subtly influence the suggestions presented to users, shaping their decision-making processes. Decision-making biases play a significant role in how AI algorithms generate recommendations tailored to individual users. These biases can stem from various factors, including user behavior data, preferences, and historical interactions. When users engage with AI-driven suggestions, they may unknowingly fall victim to biases such as confirmation bias, where they prefer recommendations that align with their existing beliefs or choices. Additionally, anchoring bias can influence users' decisions by heavily weighing initial suggestions presented to them.
User trustworthiness is crucial in combating the effects of cognitive biases in AI recommendations. Building trust between users and AI systems involves transparency in how recommendations are generated and allowing users some control over the recommendation process. By enhancing user trustworthiness, organizations can mitigate the impact of biases and provide more accurate and relevant recommendations to their users. As AI continues to evolve, understanding and addressing decision-making biases becomes increasingly vital in optimizing user experiences and outcomes.
User Engagement Through Personalized Content
User engagement amplifies through the delivery of personalized content tailored to individual preferences and behaviors. This personalized approach enhances user interactions and fosters a deeper connection with the content provided.
Here's how this strategy enhances engagement:
- Visual Imagery: Imagine a user scrolling through a website where each product recommendation is tailored to their past purchases and browsing history, creating a seamless and personalized shopping experience.
- Behavioral Triggers: As the user navigates through different sections, the content dynamically adjusts based on their real-time interactions, increasing relevance and engagement.
Ethical Considerations in AI Recommendation Systems
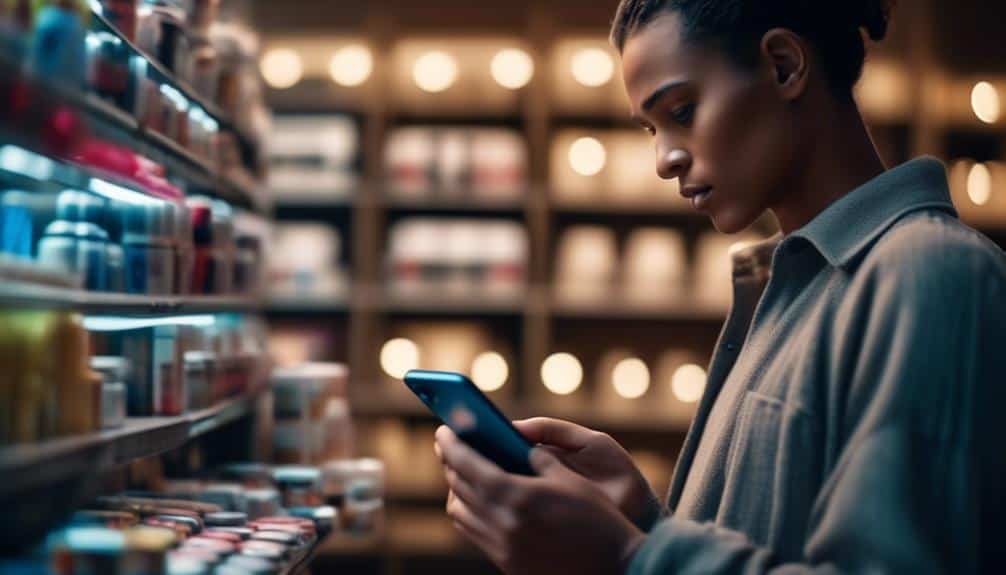
Enhancing user engagement through personalized content raises critical ethical considerations in AI recommendation systems. Data privacy is a key concern as these systems collect vast amounts of user data to tailor recommendations. Ensuring transparency in algorithms is crucial to building trust with users and safeguarding their information. Detecting biases within AI algorithms is essential to prevent discriminatory outcomes in recommendations. Implementing fairness frameworks can help mitigate biases and ensure equitable treatment for all users.
To address ethical considerations effectively, companies must prioritize data privacy by implementing robust security measures and obtaining user consent for data usage. Algorithm transparency can be achieved through clear explanations of how recommendations are generated, empowering users to understand and trust the system. Proactive bias detection mechanisms and fairness frameworks should be integrated into AI recommendation systems to uphold ethical standards and promote inclusivity.
Frequently Asked Questions
How Do AI Recommendation Systems Handle User Data Privacy and Security Concerns?
When it comes to user data privacy and security concerns, AI recommendation systems prioritize data encryption to safeguard information. They also adhere to strict privacy regulations to ensure compliance and build trust with users.
What Are the Potential Drawbacks of Relying Solely on AI Recommendations for Customer Decision-Making?
Relying solely on AI recommendations for customer decision-making can have ethical implications. User trust may erode if personal preferences are misunderstood or if biases in the data used for recommendations are not addressed.
How Do AI Recommendation Systems Take Into Account Cultural Differences and Individual Preferences?
AI recommendation systems leverage advanced algorithms to analyze cultural nuances and individual preferences. By collecting and analyzing vast amounts of data, these systems create personalized experiences that cater to diverse backgrounds and unique tastes seamlessly.
What Measures Are in Place to Prevent Algorithmic Bias and Ensure Fair and Unbiased Recommendations?
To ensure unbiased recommendations, ethical implications are considered, biases are mitigated through algorithm transparency, and user feedback is utilized. These measures promote fairness and accountability in AI systems, fostering trust and reliability.
How Do AI Recommendation Systems Adapt to Changing Customer Behavior and Preferences Over Time?
To adapt to changing customer behavior, AI recommendation systems utilize behavior tracking for personalized recommendations. Through continuous learning, these systems make dynamic adjustments, ensuring tailored suggestions based on evolving preferences and habits over time.
Conclusion
In conclusion, the psychology behind AI recommendations is a complex interplay of data, user preferences, emotions, cognitive biases, and ethical considerations.
By leveraging behavioral insights and personalization, businesses can enhance customer experience and drive user engagement.
Understanding the underlying psychological mechanisms behind AI recommendations is crucial in delivering tailored content that resonates with customers on a deeper level.
This data-driven approach can lead to more effective and impactful recommendation systems in the future.