AI and the Pulse of Social Platforms: Identifying Trends in Real-Time
In the vast sea of social platforms, AI serves as the lighthouse guiding you through the waves of data, illuminating trends as they crest and fall.
Imagine having the ability to navigate the ever-changing currents of online conversations in real-time, spotting emerging patterns before they make a splash.
As you explore the intricate dance between artificial intelligence and the dynamic landscape of social media, you'll uncover how algorithms sift through the digital chatter to reveal the heartbeat of trending topics.
The fusion of technology and human interaction paints a fascinating picture of the pulse of society, and the insights awaiting you in this realm are as intriguing as they are impactful.
Key Takeaways
- AI plays a pivotal role in swiftly identifying real-time patterns and shifts on social platforms.
- Real-time data collection and analysis tools provide instantaneous access to current information for quick adaptation to market dynamics.
- Sentiment analysis and emotion detection techniques help in gauging user reactions and tailoring strategies based on sentiment analysis.
- Trend prediction powered by machine learning algorithms offers reliable insights for businesses to make data-driven decisions and engage with personalized content.
The Role of AI in Trend Analysis
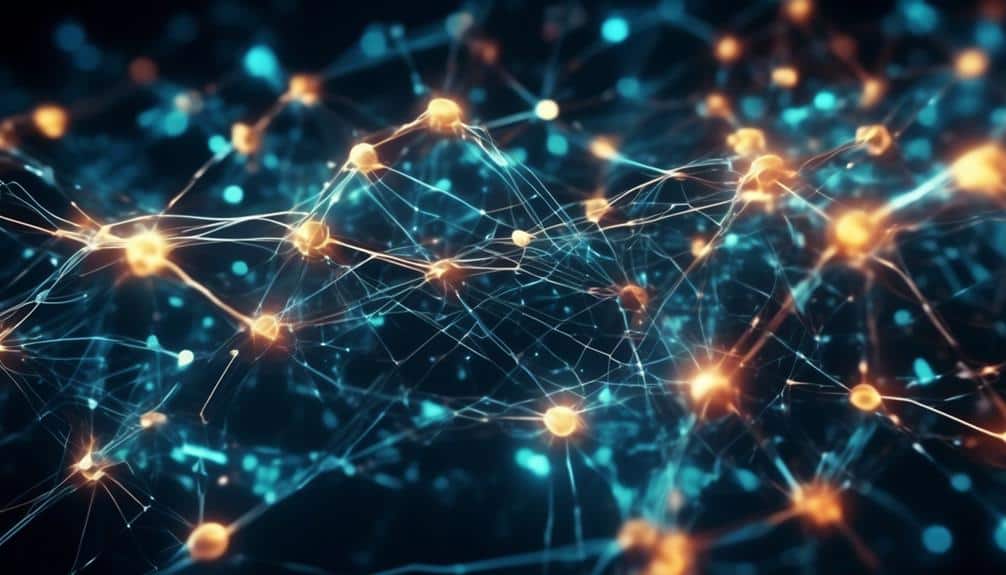
In trend analysis, AI plays a pivotal role in swiftly identifying real-time patterns and shifts in social platforms. AI-driven pattern recognition enables machines to sift through vast amounts of data with unparalleled speed and accuracy, providing insights that human analysts may overlook. Trend forecasting algorithms powered by AI can detect emerging patterns, allowing businesses to adapt quickly to changing market dynamics.
By leveraging AI in trend analysis, companies gain a competitive edge by anticipating shifts in consumer behavior and market trends. These algorithms can analyze social media posts, search queries, and online interactions to identify patterns that indicate upcoming trends. The ability of AI to process massive datasets in real-time enables businesses to make data-driven decisions swiftly and stay ahead of the curve.
AI-driven pattern recognition is transforming the way trend analysis is conducted, providing businesses with actionable insights to drive strategic decisions. By utilizing trend forecasting algorithms powered by AI, organizations can proactively respond to market trends and capitalize on emerging opportunities in the dynamic landscape of social platforms.
Real-Time Data Collection Methods
Real-time data collection methods enhance the efficiency of trend analysis by providing instantaneous access to current information from social platforms. Real-time monitoring tools play a crucial role in capturing and analyzing data as it unfolds, allowing for immediate insights into emerging trends. By leveraging real-time monitoring, businesses can stay ahead of the curve by identifying shifts in consumer behavior, market sentiments, and viral content almost as soon as they occur.
Data visualization is a key component of real-time data collection methods, enabling users to interpret complex datasets quickly and make informed decisions. Through interactive charts, graphs, and dashboards, data visualization tools transform raw data into visual representations that highlight patterns, anomalies, and correlations in real-time. This visual representation not only simplifies the analysis process but also facilitates rapid decision-making based on the latest trends observed on social platforms.
Incorporating real-time monitoring and data visualization techniques into trend analysis empowers businesses to adapt swiftly to changing market dynamics, capitalize on emerging opportunities, and maintain a competitive edge in the fast-paced digital landscape.
Sentiment Analysis for Trend Detection
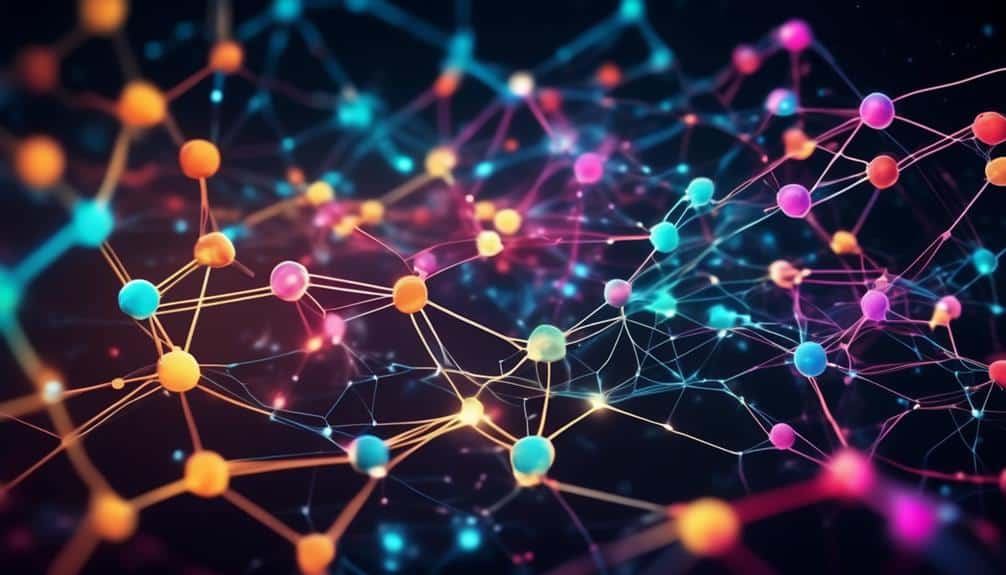
When analyzing sentiment for trend detection, you'll focus on emotion detection techniques to gauge the overall mood of a topic. This approach plays a crucial role in enhancing the accuracy of trend prediction by incorporating the sentiment of the discussions into the analysis.
Emotion Detection Techniques
Implementing sentiment analysis techniques for emotion detection in AI-driven trend identification allows for real-time tracking and analysis of user reactions on social platforms. By integrating advanced technologies like facial recognition and behavioral analysis, AI can now accurately interpret emotions expressed in text, images, and videos shared online.
These techniques enable the identification of trends driven by specific emotional responses, providing valuable insights for marketers, researchers, and platform developers. Emotion detection algorithms can distinguish between varying sentiments such as joy, anger, or sadness, allowing for a more nuanced understanding of user engagement.
This level of granularity in analyzing emotions in real-time empowers businesses to tailor their strategies effectively, respond promptly to emerging trends, and engage with their audience in a more personalized manner.
Trend Prediction Accuracy
By leveraging sentiment analysis techniques for emotion detection in AI-driven trend identification, a heightened focus on trend prediction accuracy emerges through the nuanced understanding of user engagement based on distinct emotional responses.
- Accuracy Assessment: Utilizing advanced algorithms to measure the precision of trend predictions.
- Data Visualization: Presenting trend forecasting results in visually compelling formats for easy interpretation.
- Trend Forecasting: Implementing cutting-edge methodologies to anticipate upcoming trends with greater reliability.
- Performance Evaluation: Assessing the effectiveness of trend prediction models through rigorous testing and analysis.
Through continuous refinement of sentiment analysis models and enhanced data processing capabilities, achieving higher accuracy in trend prediction remains a primary objective in the evolution of AI-driven social platform analytics.
Machine Learning Algorithms in Action
Machine learning algorithms actively drive the real-time trend identification process on social platforms. Through advanced image recognition capabilities, these algorithms sift through vast amounts of visual data to uncover emerging trends. By analyzing patterns in images shared across platforms, they can quickly identify popular themes, products, or events.
Additionally, machine learning algorithms power personalized recommendations, enhancing user experiences by suggesting content tailored to individual preferences. These algorithms learn from user interactions, continuously adapting to provide relevant and engaging suggestions. As a user, you benefit from these algorithms' ability to analyze vast datasets in real-time, presenting you with content that aligns with your interests.
The seamless integration of image recognition and personalized recommendations showcases the power of machine learning in shaping the social platform experience. Stay tuned for further advancements as these algorithms continue to evolve and enhance trend identification processes.
Social Listening Tools and Techniques
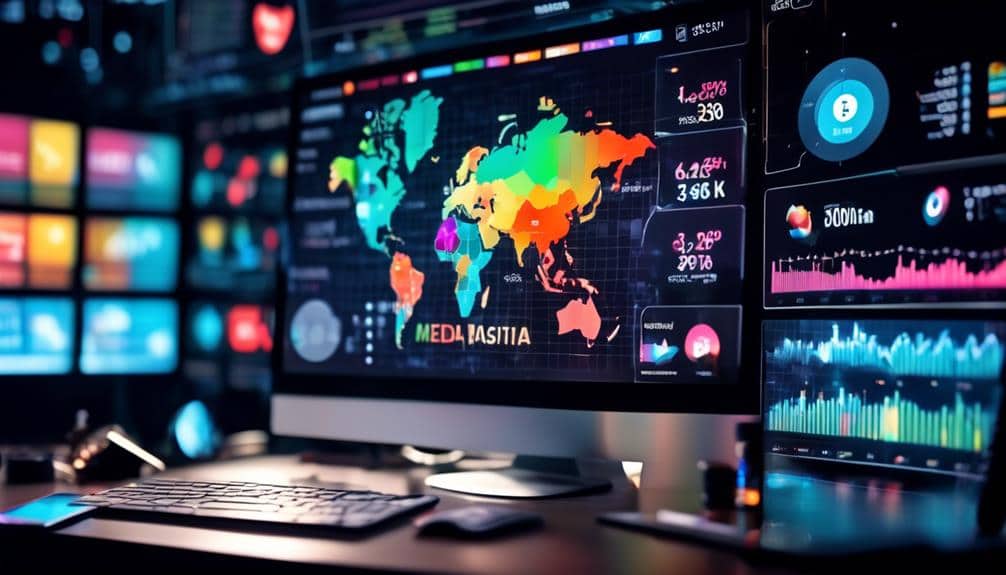
Drawing insights from the data analyzed by machine learning algorithms, social listening tools and techniques offer a deeper understanding of user sentiments and interactions across digital platforms. These innovative tools leverage cutting-edge technologies to provide real-time analytics and valuable insights.
Here are four key aspects highlighting the significance of social listening tools and techniques:
- Image Recognition: Advanced algorithms now enable social listening tools to analyze images shared on platforms, allowing for a more comprehensive understanding of user behavior and preferences.
- Social Media Monitoring: By constantly monitoring social media platforms, these tools can track trends, brand mentions, and consumer feedback in real-time, enabling businesses to adapt quickly to changing market dynamics.
- Sentiment Analysis: Through natural language processing, social listening tools can gauge the sentiment behind user-generated content, providing valuable insights into public opinion and brand perception.
- Competitor Analysis: These tools offer the ability to track competitors' activities and strategies, allowing businesses to benchmark their performance and identify areas for improvement.
Leveraging Natural Language Processing
Leveraging Natural Language Processing enhances social listening tools' capacity to extract valuable insights from user-generated content with precision and efficiency. By incorporating advanced techniques such as semantic analysis and chatbot integration, organizations can gain a deeper understanding of online conversations and trends in real-time.
Leveraging Natural Language Processing in Social Listening Tools
Technique | Description |
---|---|
Semantic Analysis | Examines the meaning behind words to understand context and sentiment. |
Chatbot Integration | Incorporates AI-powered chatbots to engage with users and extract valuable data efficiently. |
Semantic analysis allows for the interpretation of language nuances, enabling platforms to identify sentiment, intentions, and emerging topics accurately. Moreover, chatbot integration streamlines data collection processes by automating interactions with users, ensuring a continuous flow of information. This integration of Natural Language Processing techniques revolutionizes social listening, providing organizations with actionable insights to drive strategic decision-making.
Predictive Analytics for Future Trends
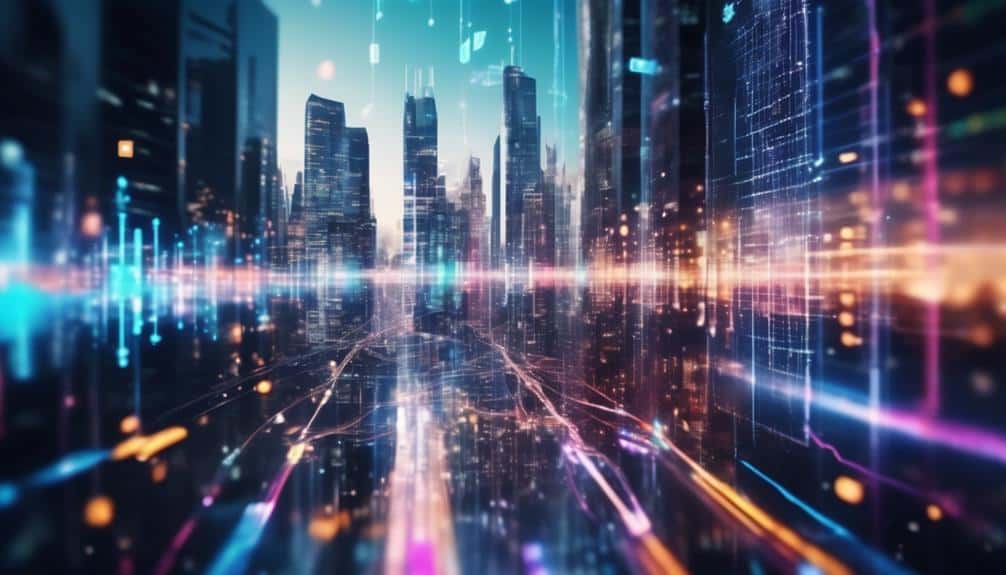
Utilizing predictive analytics enables organizations to forecast future trends based on data-driven insights, enhancing strategic planning and decision-making processes. By incorporating advanced algorithms and machine learning models, predictive analytics can provide valuable foresight into upcoming developments.
Here are four key aspects to consider when delving into predictive analytics for future trends:
- Data Visualization Techniques: Utilize interactive graphs and charts to represent complex data sets visually, allowing for easier interpretation and understanding of trends.
- User Behavior Analysis: Analyze user interactions and patterns to predict future preferences and behaviors accurately.
- Pattern Recognition: Identify recurring trends and anomalies within data sets to anticipate potential outcomes.
- Predictive Modeling: Develop models that can simulate future scenarios based on historical data, enabling proactive decision-making.
Case Studies on Trend Prediction
As organizations harness predictive analytics for future trends, real-world case studies offer invaluable insights into the effectiveness of trend prediction methodologies. Trend forecasting accuracy assessment is crucial in evaluating the reliability of predictive models, especially in the dynamic realm of social media and cultural shifts. Let's delve into two case studies that exemplify the significance of trend prediction in today's fast-paced digital landscape.
Case Studies on Trend Prediction
Case Study | Industry | Trend Prediction Outcome |
---|---|---|
Fashion Retailer | E-commerce | Successfully forecasted a surge in demand for sustainable fashion, leading to a strategic shift in product offerings. |
Streaming Platform | Entertainment | Utilized sentiment analysis to predict viewer preferences, resulting in the creation of highly targeted content that boosted user engagement. |
Tech Startup | Technology | Leveraged social media data to predict emerging trends, enabling proactive development of innovative solutions ahead of competitors. |
Ethical Considerations in AI Trend Monitoring
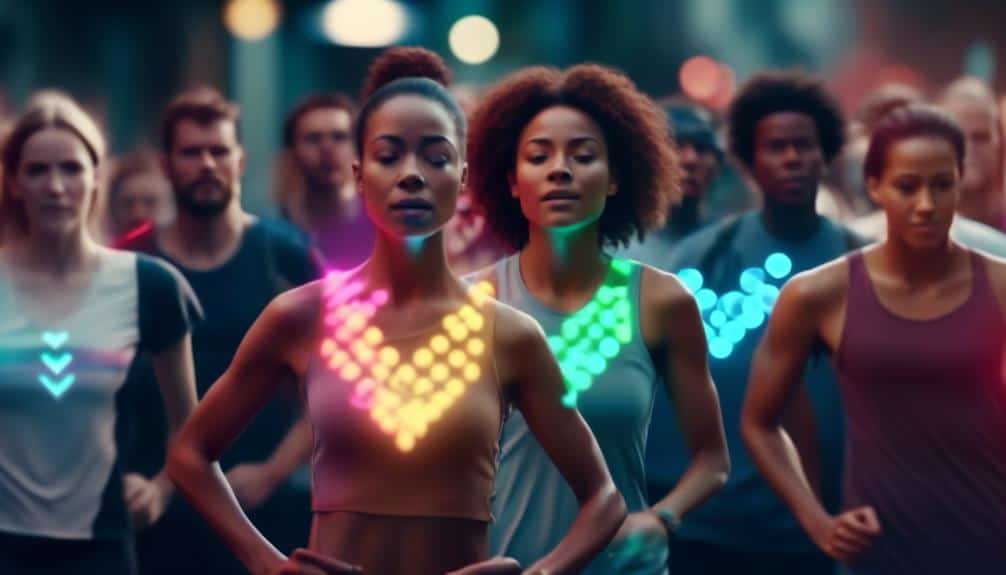
When considering ethical implications in AI trend monitoring, it's crucial to address issues surrounding privacy and data protection. Detecting and mitigating biases in AI algorithms is another key aspect to ensure fair and accurate trend analysis.
Privacy in AI Monitoring
Privacy considerations in AI monitoring are paramount due to the potential ethical implications of tracking real-time trends. When engaging in this practice, it's crucial to prioritize data privacy and ensure that user consent is obtained. Here are key aspects to focus on:
- Data Encryption: Implement robust encryption protocols to safeguard sensitive information.
- Anonymization Techniques: Utilize methods to anonymize data and protect individual identities.
- Access Controls: Restrict access to AI monitoring systems to authorized personnel only.
- Transparent Policies: Clearly communicate privacy policies to users, detailing how their data is collected and used.
Bias Detection in AI
Implementing bias detection mechanisms in AI trend monitoring is crucial to ensure ethical considerations are upheld. Fairness assessment plays a key role in identifying and mitigating biases that may skew the results of trend monitoring algorithms.
By conducting regular fairness assessments, you can ensure that the AI algorithms are treating all data fairly and without discrimination. Algorithm transparency is equally vital in bias detection, as it allows for a deeper understanding of how decisions are being made within the AI system.
Transparent algorithms enable you to trace back any biases detected to their source, facilitating the necessary adjustments to promote unbiased trend monitoring. Prioritizing fairness assessment and algorithm transparency is fundamental in maintaining the ethical integrity of AI trend monitoring processes.
Frequently Asked Questions
How Can Businesses Effectively Leverage AI for Trend Analysis on Social Platforms?
To effectively leverage AI for trend analysis on social platforms, businesses must integrate advanced AI applications into their strategies. This allows for real-time data processing, trend identification, and informed decision-making, enhancing overall performance and competitiveness.
What Are Some Common Challenges Faced When Collecting Real-Time Data for Trend Analysis?
When collecting real-time data for trend analysis, common challenges include ensuring data accuracy and dealing with time sensitivity. Visualizing data effectively is crucial for obtaining predictive insights that can drive informed decision-making in dynamic environments.
How Can Sentiment Analysis Be Used to Detect Emerging Trends on Social Platforms?
To detect emerging trends on social platforms, sentiment analysis can classify user opinions as positive, negative, or neutral. This classification helps in trend detection by identifying shifts in sentiment towards certain topics, products, or events.
Can You Provide Examples of Machine Learning Algorithms Commonly Used in Trend Analysis?
When analyzing trends, you can select algorithms like Random Forest or Gradient Boosting for accuracy. Ensure proper feature engineering and data preprocessing for model evaluation. These steps are crucial in identifying patterns effectively.
What Are Some Best Practices for MAIntAIning Ethical Standards When Using AI for Trend Monitoring on Social Platforms?
To maintain ethical standards in AI trend monitoring on social platforms, ensure transparency by disclosing data sources and methodologies. Avoid bias through diverse training data and regular audits. Uphold privacy regulations and offer clear opt-out options.
Conclusion
In conclusion, AI plays a crucial role in analyzing trends in real-time on social platforms. By utilizing machine learning algorithms, sentiment analysis, and predictive analytics, businesses can stay ahead of the curve and make informed decisions.
Social listening tools and natural language processing further enhance trend detection capabilities. However, ethical considerations must be taken into account when monitoring trends using AI technologies to ensure data privacy and transparency.